Out of all the skills involved in qualitative research, it is analysis where most researchers want to see AI tools make the most progress. One of the biggest challenges in qual is having to process so much unstructured content within increasingly compressed timelines. AI analysis tools can instantly summarise what is said, from vast volumes of text and super-charge verbatim searches.
Since generative AI is fueled by Large Language models and language is the currency of qual research - there is a high degree of competency in how AI can extract meaning from spoken word or written transcripts. In my view AI summary tools can supplement and support but not necessarily replace human judgement. We still need the human in the loop to decide what really matters.
How AI tools impact qual analysis - video
AI is better at summaries than insight
I think it’s important to make a distinction between a summary and an insight. For me a summary is a description of what was said. This is what a novice qual researcher might do and it’s more reportage rather than interpretation. Whereas an insight is an interpretation of what people think, feel and do which is articulated in such a way that it points towards a useful recommendation. Or as Jeremy Bullimore said: ‘An insight is like a fridge - once you open the door, a light comes on’. A great insight will shine a light on a particular course of action and it will inspire ideas. An insight selectively embraces one of many possible realities and discards the alternatives. It has to be valid, well articulated and properly supported. It’s only good if it’s ultimately useful for the user of that research!
So while AI summaries can quickly tell you what was said it can’t necessarily quickly tell you what it means for the client or what matters most.
It could also miss something of critical importance. To get the insight from the data you really need a human to curate what matters and what doesn’t.
Is there a single version of reality?
At a philosophical level, in many cases I don’t believe there's a single valid interpretation in qual. It’s not as if there’s a single reality or truth. Reality is complex, subjective and open to interpretation. So it’s unlikely that AI can give you a single version of reality that happens to fuel the optimal recommendation and client decision, when there’s so many competing versions of reality. For me the most valuable skill of a qual researcher is in this curation of meaning - cutting through all the data and ignoring multiple competing interpretations and then honing in on the single version of reality that really matters and will inspire the optimal decisions. This relies on the strategic ability of the researcher (and the quality of the briefing).
Acceleration of substantiation
In my view, the main current benefit in AI summary tools for typical qual research study designs (like focus groups, depths, mobile ethnography and asynchronous text based studies) is the acceleration of substantiation, rather than discovering the story in the first place. AI summary tools allow you to scrutinise qual data more quickly and at a larger scale (without necessarily having to trudge through all the transcripts word for word). They quickly generate summaries of the themes in the text and remind you of things a moderator may have forgotten, which improves quality of output.
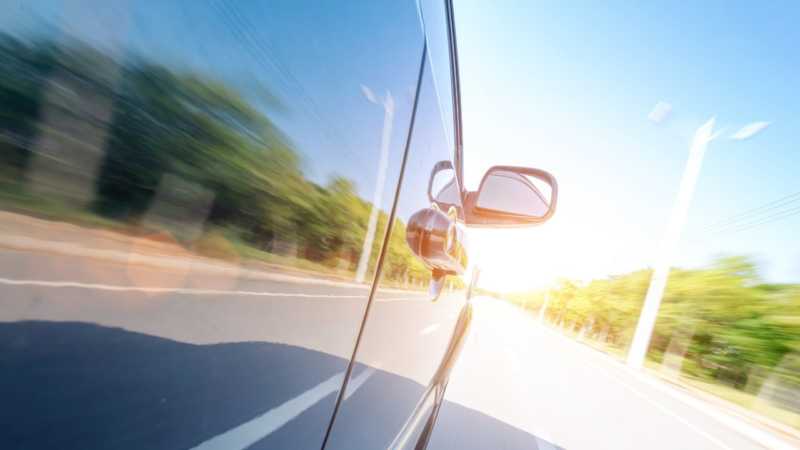
I think AI summary tools are more effective in the hands of the person who moderated the study in the first place - since they already have a strong idea of the most strategically relevant story. So they can use the AI tools to test, develop and stretch their hypotheses. It’s less likely that the AI summary will uncover an insight that the human researcher didn’t consider - although it can play a useful role as a counter balance - stretching the researcher beyond their own biases.
Humans should control the narrative
The expert human researcher in the loop is still valuable. That’s partly because each client and their brief is unique and the optimal story of analysis will depend on many factors that are simply not in AIs learning data nor realistically in the user’s prompts. For example, AI will struggle to factor in all that the client knows (and doesn’t know), the stakeholders’ political situation, the broader current cultural context, what can and can’t be executed, what feels emotionally salient or creatively inspiring, and so on. Whereas the human researcher will know much of this both explicitly and intuitively, taking into account the unspoken or unwritten, when they craft and articulate appropriate, strategic narratives.
In many ways the better someone is at qual research, the better they will be at use AI analysis tools. They can work out the right questions to ask of the data - just as they do in human-only analysis.
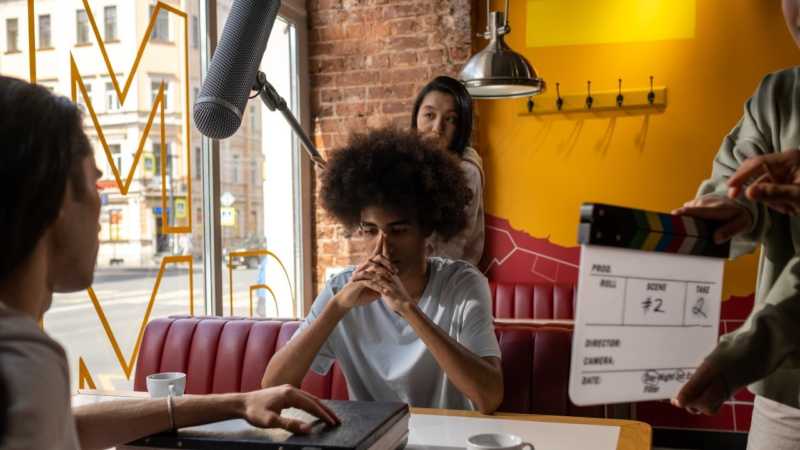
Different briefs require different depth
Whether AI summary tools are good enough (and how much human intervention they need) depends on the context of the brief. If people doing research over-rely on AI summaries which over simplify or miss critical details then they’ll be performing worse than a more human intensive approach. That said, if their summaries are good enough and the brief is relatively straightforward they can offer “good enough” top level summaries - and so ultimately represent a faster track to basic findings.
Qual at scale
AI also enables qual at scale - which refers to larger, quant-esque sample sizes with qual-like open-questions and automated probes. To those in procurement this may look like a better cost per head compared to traditional human intensive qual. No doubt it will uncover insights that a quant study - with its more closed lines of questioning - may not.
So qual at scale can represent a more open version of quantitative research. But ultimately for me, the real benefit in qual is its depth.
Qual is predicated on the assumption that careful recruitment of representative sample can reveal insights which can be extrapolated to a larger population. I think the true power of qual is in speaking to less people in more depth rather than the other way around.
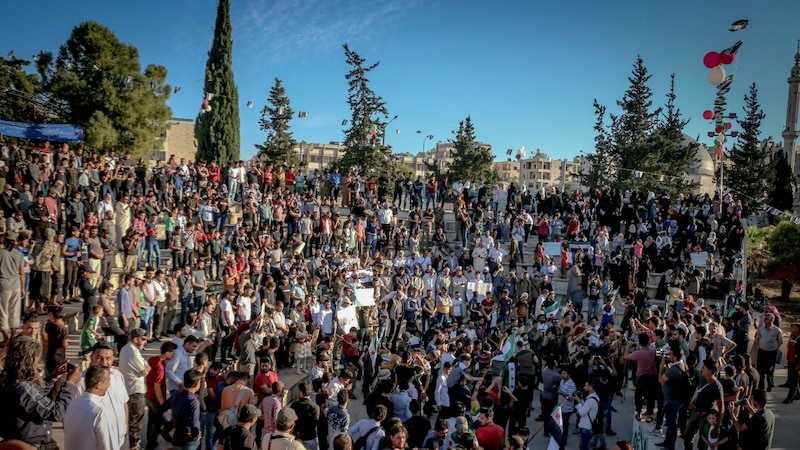
The lifeblood of qual is authenticity
We should not forget that the lifeblood of qual is authenticity. So if we blindly follow AI summaries without due diligence and without insisting on transparency by which I mean the need to check conclusions against the source data - qual research’s reputation could suffer. While I’ve not seen much evidence of the much lamented hallucinations that generative AI can do - it only takes one hallucination or error in a report to quickly lose integrity.
Can AI make recommendations?
I must say Chat GPT is also impressive at making recommendations. The tighter the insights you feed it the more sensible its suggestions on what means for the client. However again, I see AI recommendations as more food for thought rather than a valid substitute for expertise.
I’m excited by the progress in this space although I urge people doing research to go back to the data when they can to ensure they’re capturing the true gold in what was said.